A Guide To Training
Chatbots
Explore the data sources that make an AI chatbot into a brilliant genius for your business!
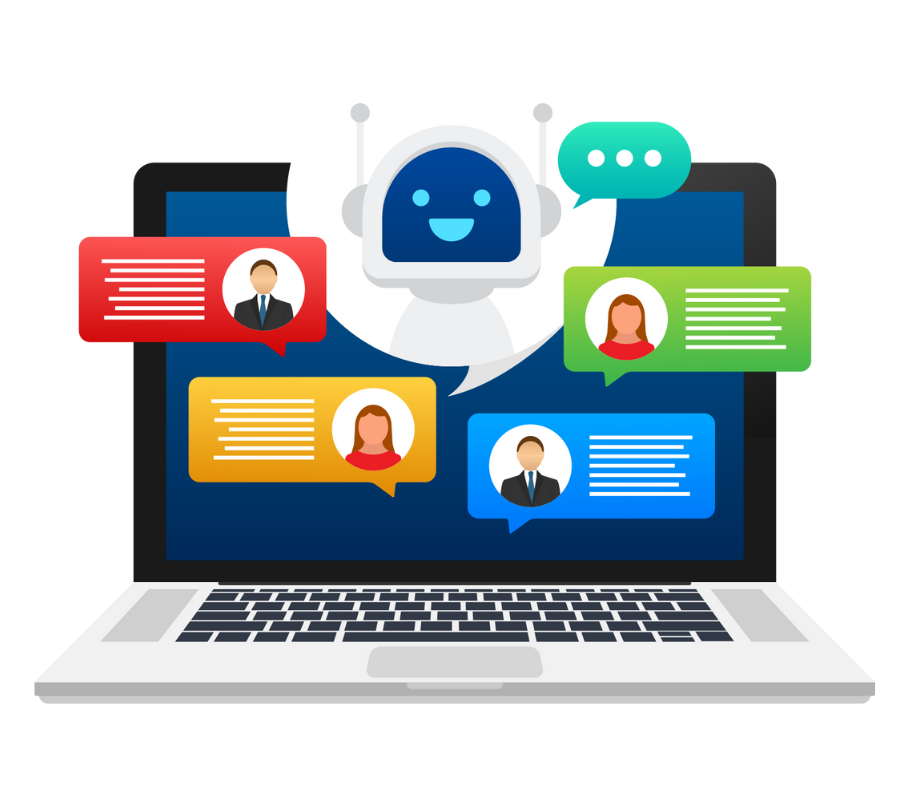
Explore the data sources that make an AI chatbot into a brilliant genius for your business!
Explore the data sources that make an AI chatbot into a brilliant genius for your business!
Explore the data sources that make an AI chatbot into a brilliant genius for your business!
In recent years, using artificial intelligence-powered software programs to automate customer service and other tasks has become increasingly popular. One such system, a chatbot, can communicate with customers in an interactive and natural way—allowing them to get quick responses to their inquiries without waiting for a human employee.
However, the release of ChatGPT and particularly its increasingly sophisticated large language model, has created a newfound appreciation of the potential use cases beyond customer service. With AI, the sky is truly the limit. Understanding how AI chatbots work and how they are trained can help businesses brainstorm and identify potential opportunities or use cases. Keep in mind that the chat interface—whether based on text or voice—is the mechanism that delivers the experience. Just like with automobiles, the engine that underpins the shiny exterior is what delivers the true value.
By definition, a chatbot is a computer program that can simulate conversation with humans using natural language processing (NLP) techniques. The chatbot’s programming uses rules-based or self-learning algorithms to understand what the customer asks for, quickly giving accurate responses.
Generally speaking, there are two types of chatbots: rule-based bots that follow predefined user commands, and more advanced AI bots that can learn from each conversation. Rule-based chatbots have limitations. Its conversations are basic because it’s training is based on “if/then” conditions. So, if the chatbot is asked X, it must respond Y. Rule-based chatbots are simpler, faster to train, and less expensive. But they are also far less fluid or expansive.
AI chatbots, on the other hand, use machine learning to grasp the meaning and context of the question/prompt to generate a response. Additionally, because it is trained on vast datasets and continually learns, it can generate intelligent responses—including asking the user a follow-up question. The sophistication and intelligent capabilities of learning-based language models are one of the reasons why ChatGPT has become a catalyst for AI adoption.
Chatbots are usually trained through deep learning—a form of machine learning that uses large datasets to identify patterns in data points. Deep learning algorithms can process thousands of datasets and learn how to respond accurately by analyzing the context behind each query. As the bot interacts with more users over time, it continuously trains on each new conversation, improving accuracy with every experience it gains. Additionally, some companies train their chatbot on specific data relevant to their business—such as company protocols or product catalogs—to give users tailored responses that fit their needs.
To maximize the effectiveness of a chatbot, companies must train the AI system properly. By leveraging available data sets relevant to their business, companies can ensure that their chatbot provides accurate responses tailored to their user's needs—resulting in better results and overall experience.
To maximize the effectiveness of a chatbot, companies must train the AI system properly. By leveraging available data sets relevant to their business, companies can ensure that their chatbot provides accurate responses tailored to their user’s needs—resulting in better results and overall experience.
Training data can take the form of structured and unstructured data. Structured data is organized and formatted in a consistent order so that it’s easy to read and comprehend (e.g., quantitative data, phone numbers, email addresses, etc.). Unstructured data, on the other hand, is not organized in a predefined way and needs to be analyzed and interpreted. Unstructured data include open-ended text, social media posts, customer reviews, emails, videos, audio files, and more.
Let’s Talk. We’ll help you take the next leap forward.
Data sources for chatbots can be diverse, so it’s important to consider the possibilities with the limitations. The accuracy of chatbot outputs is highly dependent on the quality of the data used to train them. While chatbots cannot currently discern fact from fiction, they can still be highly effective tools when used with vetted and high-quality data.
It’s worth noting that human oversight is necessary to ensure that data is accurate and free from biases that could result in misleading or incorrect results. With that in mind, many data sources can be used to train chatbots for both public-facing and internal applications.
Customer support is one of the common use cases for chatbots. Customer support queries are among the most effective data sources to train a chatbot. Such sources include FAQs from previous customers and conversations between customer service representatives and customers—allowing the bot to understand the context behind inquiries and generate accurate responses. As the bot interacts with more users over time, it learns from these conversations and improves accuracy.
Learn how the Quantilus team developed a highly customized deep-learning model capable of complex, high-volume queries and personalized responses for a financial services client.
Learn how the Quantilus team developed a highly customized deep-learning model capable of complex, high-volume queries and personalized responses for a financial services client.
Another key item for tailoring chatbot responses is feeding it data related to company products. Examples include catalogs, datasheets, metadata, inventory, or anything associated with the product. For example, a clothing retailer may choose to train its bot on its inventory so that customers can quickly search for items by typing keywords or selecting filters such as color or size. Also, suppose a particular product isn’t available in the store now. In this case, the bot could suggest similar items available online or in another location near them. In this way, businesses can ensure that all customer requests are responded to accurately and promptly, improving loyalty and satisfaction scores overall. This same chatbot could also demonstrate use cases internally, affording employees real-time access to product information for their various functions (e.g., marketing, sales, customer service, procurement, etc.).
By leveraging AI algorithms such as sentiment analysis tools or predictive analytics systems, companies can gain valuable insights into customer behaviors and preferences—allowing businesses to tailor product recommendations based on individual shopping patterns or optimize campaigns according to buyer trends. Furthermore, companies can track customer feedback, such as ratings and reviews regarding specific products—enabling businesses to identify areas where improvements are needed.
Chatbots could come in handy at companies with hundreds or thousands of employees. For example, internal chatbots can be used for HR support. Such chatbots can answer common queries about benefits and vacation time and provide employees with information about current and upcoming company events or company policies. Standard operating procedures, department processes, or organizational directories are potential data sources that can train the chatbot to respond to “how to” requests or connect people within the organization for greater productivity. By automating these types of inquiries, companies can save valuable time and resources while ensuring employees have access to the information they need quickly and accurately.
Chatbots can be trained to understand the nuances of contracts and leases, providing a valuable resource for organizations. With AI-driven capabilities for understanding the details of complex agreements, chatbots can provide insight into potential risks or opportunities associated with various contractual arrangements. Additionally, chatbots can help streamline processes related to contract negotiation and management by automatically answering common questions about your company’s terms and conditions. In addition to risk analysis, chatbots are capable of synthesizing historical data from legal documents such as pricing or payment amounts that provide insights for those within your company that may be negotiating a new deal with a vendor. By automating and simplifying complex tasks, chatbots can provide a range of benefits that save time and resources while helping companies stay compliant with applicable regulations.
Reference materials like encyclopedias, textbooks, handbooks, dictionaries, almanacs, statistics, and more could train the chatbot. Research in the form of scholarly articles, research reports, academic articles, dissertations, and theses could train a chatbot. For example, companies creating reference materials could “productize” their entire catalog of materials into a chatbot (assuming said company owns the rights). And for an intra-organizational use case, a pharmaceutical company can use their research data and academic texts to train the chatbot. Such a tool would make it efficient for employees or other researchers within the same company to easily access and learn from prior studies, but it also could inform or guide future research.
Data sources don’t necessarily have to be owned sources like the ones mentioned above. Data from third-party sources like OpenAI GPT, Common Crawl, Wikipedia, IMDb, or even Yelp could be potential data sources. As with any third-party sources, permissions must be properly and legally obtained. Additionally, there may be additional terms and conditions as well as costs for certain use cases. For example, some third-party databases may not permit use in AI applications or commercial use cases. Lastly, with third-party sources, such considerations as data quality, bias, or privacy must be assessed before moving forward.
The data sources above are by no means the only training materials. Ultimately, companies should focus on the chatbot’s goal by asking, “What problem will the chatbot solve?” Being crystal clear about your intention and the chatbot’s purpose facilitates identifying or procuring potential data sources.
If you’re exploring a chatbot for your company or your customers, Quantilus can help you crystallize your idea and your requirements. Contact us today to setup a consultation.
WEBINAR