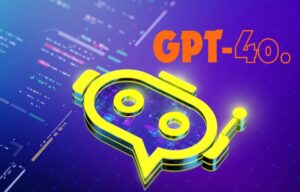
Many people have seen The Terminator and know what happens if you’re busy playing video games instead of preparing for SkyNet. That world’s artificial intelligence isn’t too far from what’s available today—but how, exactly, can AI be explained for everyone to understand?
Let’s start with its history. In 1956, Marvin Minsky and John McCarthy, who coined the term AI, described it as a task performed by a machine or program that—were a human to do the same task—would require at least some intelligence to complete. The definition has evolved somewhat in the past 68 years, but generally all AI systems include the following behaviors which we associate with human intelligence:
There are two types of AI: narrow AI and general AI. It is the narrow AI that permeates our world today, in all fields from medical to mechanic, financial to engineering, and everything in between. General AI is still a ‘pipe dream’ that computer engineers are working to creating. Most experts say we’re still a decade or two away from achieving true general AI because of its complexity.
Narrow Artificial Intelligence
Most computers use narrow AI. They’re intelligent systems that know how to conduct specific tasks without having been explicitly programmed to do so. Apple’s Siri is a perfect example. So is Amazon’s Alexis, Google’s new virtual assistant, and IBM’s Watson supercomputer.
These systems simulate a human being’s knowledge and cognitive ability within specific parameters. The systems can include self-driving cars and spam filters. Why? Because the systems use pattern recognition, natural language processing, machine learning, and data recognition to make decisions.
Narrow AI, in addition to telling you a joke or the weather, has a host of applications. These systems can identify inappropriate content online or in emails, respond to customer service requests, read video feeds from drones, organize and coordinate business/personal calendars, analyze data to make predictions, and more.
General Artificial Intelligence
This AI—also referred to a human-level, strong, and superintelligence AI—can understand and reason within its environment, just like a human. Think Data from Star Trek: The Next Generation, or Hal from 2001 A Space Odyssey.
It’s “strong” because this AI will be stronger than us humans and “general” because we’ll be able to apply it to all problems. However, it’s nearly impossible to create a computer that can think abstractly, innovate, or plan. Experts agree that it’s really difficult—at this point still impossible—to teach a computer how to invent something that doesn’t exist.
AI is gaining strength—it can produce more accurate predictions about the data it’s fed. That DeepMind algorithms can win more games and transfer learning from one game to another is another indication that AI is growing stronger.
Dr. Hiroshi Yamakawa, Director of Dwango AI Laboratory, is one of the world’s foremost authorities on AI. He says that currently, AI can solve particular issues or address specific problems. His organization is using the Whole Brain Architecture Approach which is an engineering-based approach to “create a human-like artificial general intelligence (AGI) by learning from the architecture of the entire brain.” This AGI uses the human brain’s hard wiring as a model to integrate machine learning modules and artificial neural networks. He theorizes that the WBAI will be achieved by 2030 and will help to find solutions for global problems that include environmental, food, and space issues.
Computer scientists continue to work to develop an actual AI that can think like a human. We’ve seen the “results” of such successes in Terminator, I, Robot, A.I. Artificial Intelligence, Ex Machina, Blade Runner, and many other sci-fi books movies and books.
But the reality is that even the world’s best machine learning engineers, with access to millions of dollars, are struggling to build a general AI product. Nearly $15.2B of capital venture was given to AI startups in 2017 and over 45,000 research papers on AI have been published since 2012. Read this article to learn more about what’s propelling the recent surge in general AI development.
The closest thing we’ve got today to general AI is machine learning (ML). This term describes feeding vast amounts of data into a computer system which then extrapolates it to carry out a specific task—like Facebook’s algorithms that can recognize faces from your contacts list or Waze and Google Maps, that can analyze traffic speeds and plot alternate routes. And there are many other examples of machine learning, a growing field designed to create machines that are faster and more accurate.
In a nutshell, this subset of AI uses statistical techniques to enable a computer to learn without explicit programming. According to Dr. Yoshua Bengio, from the University of Montreal, machine learning uses data, observations, and world interactions to provide computers with acquired knowledge which then facilitates the computers’ ability to accurately generalize to new settings.
ML groups a variety of algorithms by learning style or similarity in form or function. These algorithms include representation, evaluation, and optimization—their goal is to provide computers with the “skill” to interpret never-before-seen data and apply it to new situations.
The field of ML and data science continues to grow, but while these mathematical concepts can be implemented into real world applications, this so-called deep learning isn’t real intelligence… yet. It’s a type of mathematical optimization that does have limits. The “thinking” is limited to specific domains and the intelligence depends on the training dataset (so humans are still in control). It’s difficult to use it within constantly-evolving, dynamic environments and can’t be used for control problems—only classification and regression. And to ensure the greatest accuracy, it requires huge datasets.
It’s hard to say. Sixty-two years after its inception, we’re still working to achieve true AI. Weak AI systems make more and more decisions as scientists and engineers develop ways to gather, quantify, and feed more data into more algorithms.
And we must, caution Phil Torres, an Affiliate Scholar at the Institute for Ethics and Emerging Technologies, consider the human element—as AI develops, it’s incumbent upon those in the field to program human values into algorithms. After all, he says, “If we suddenly decided, as a society, that we had to solve the problem of morality—determine what’s right and what’s wrong, and feed it into a machine—in the next 20 years… would we even be able to do it?”
WEBINAR